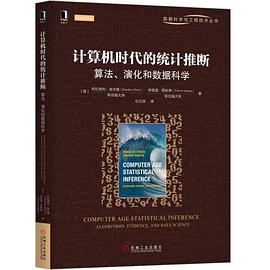
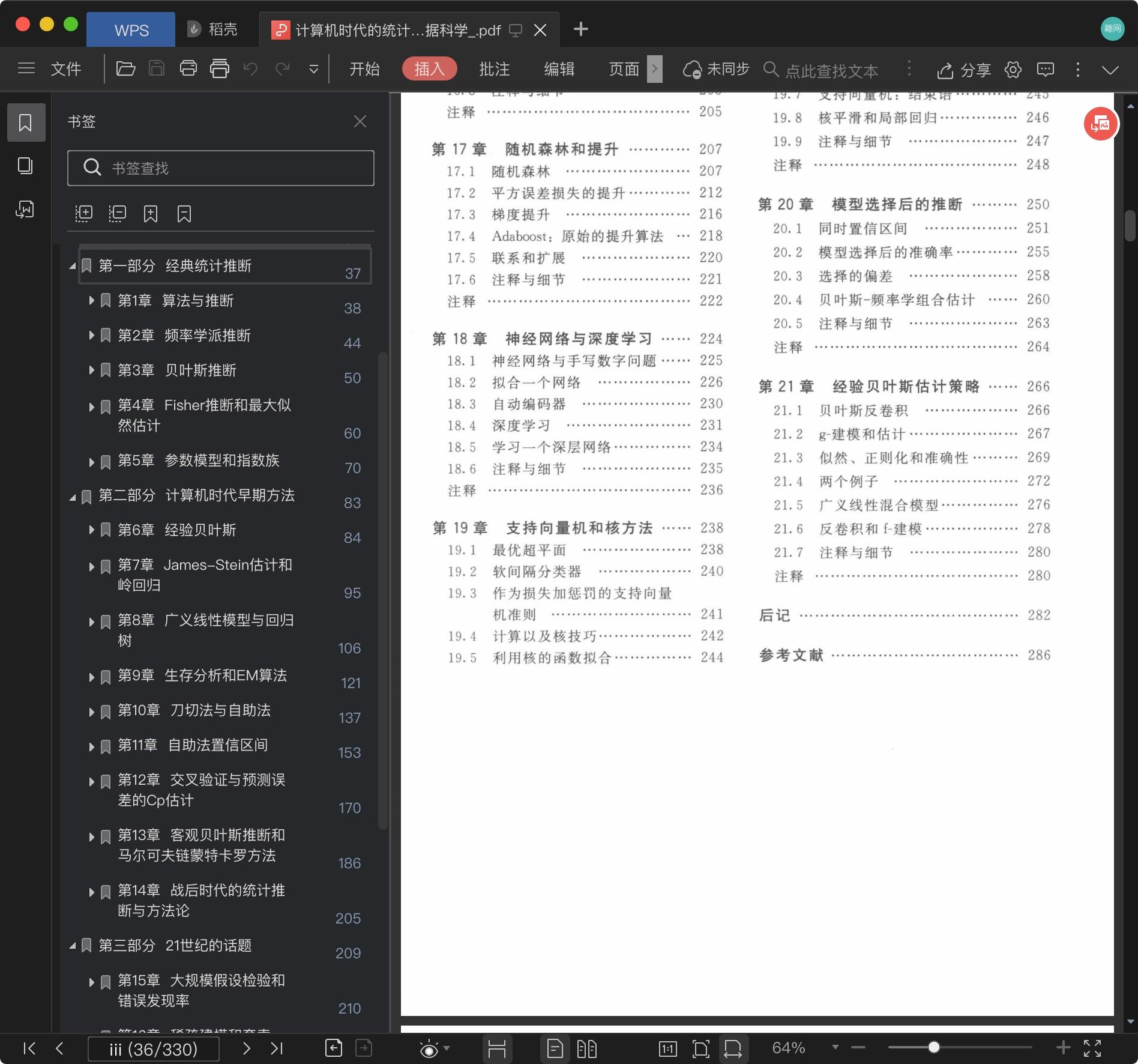
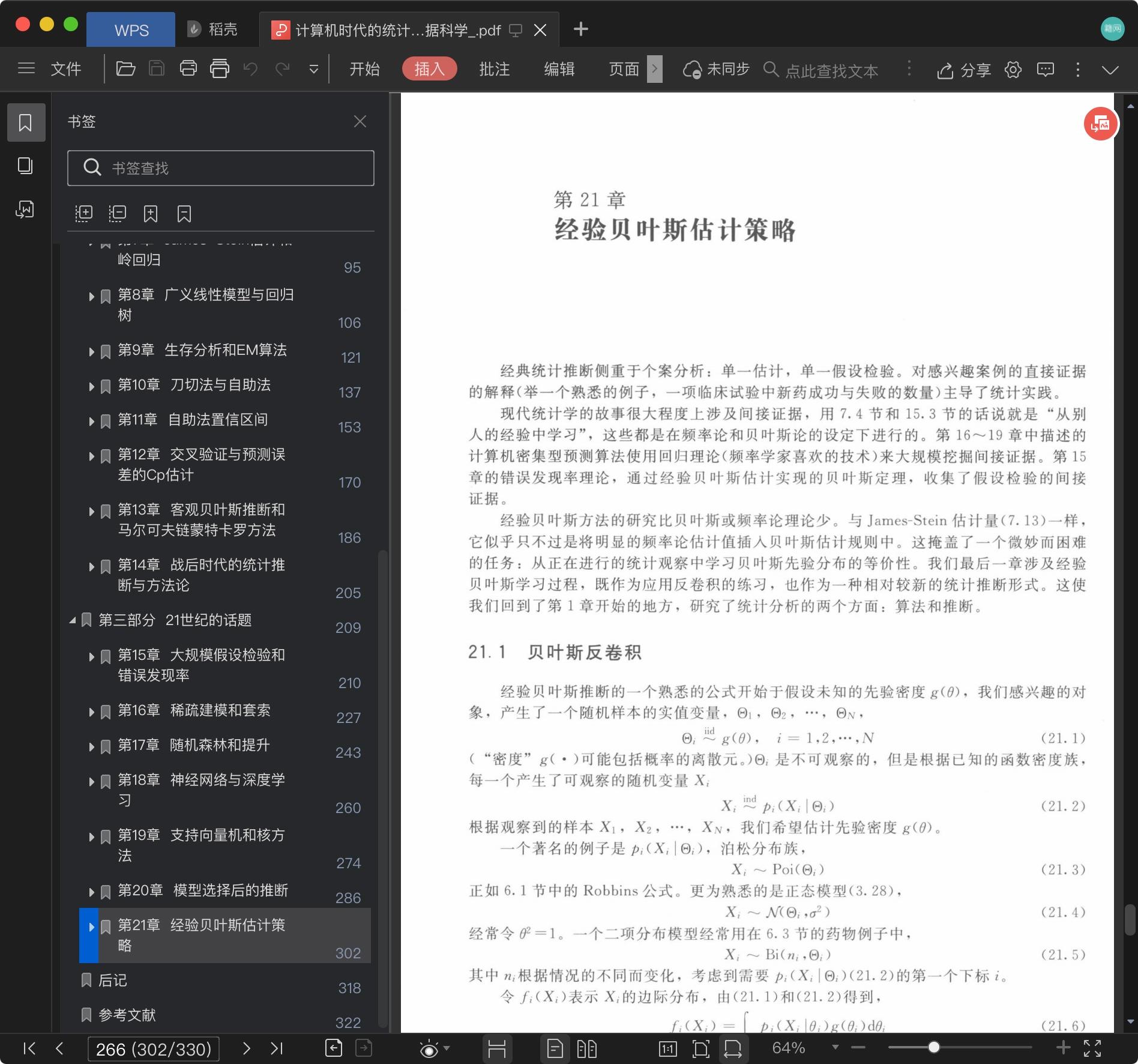
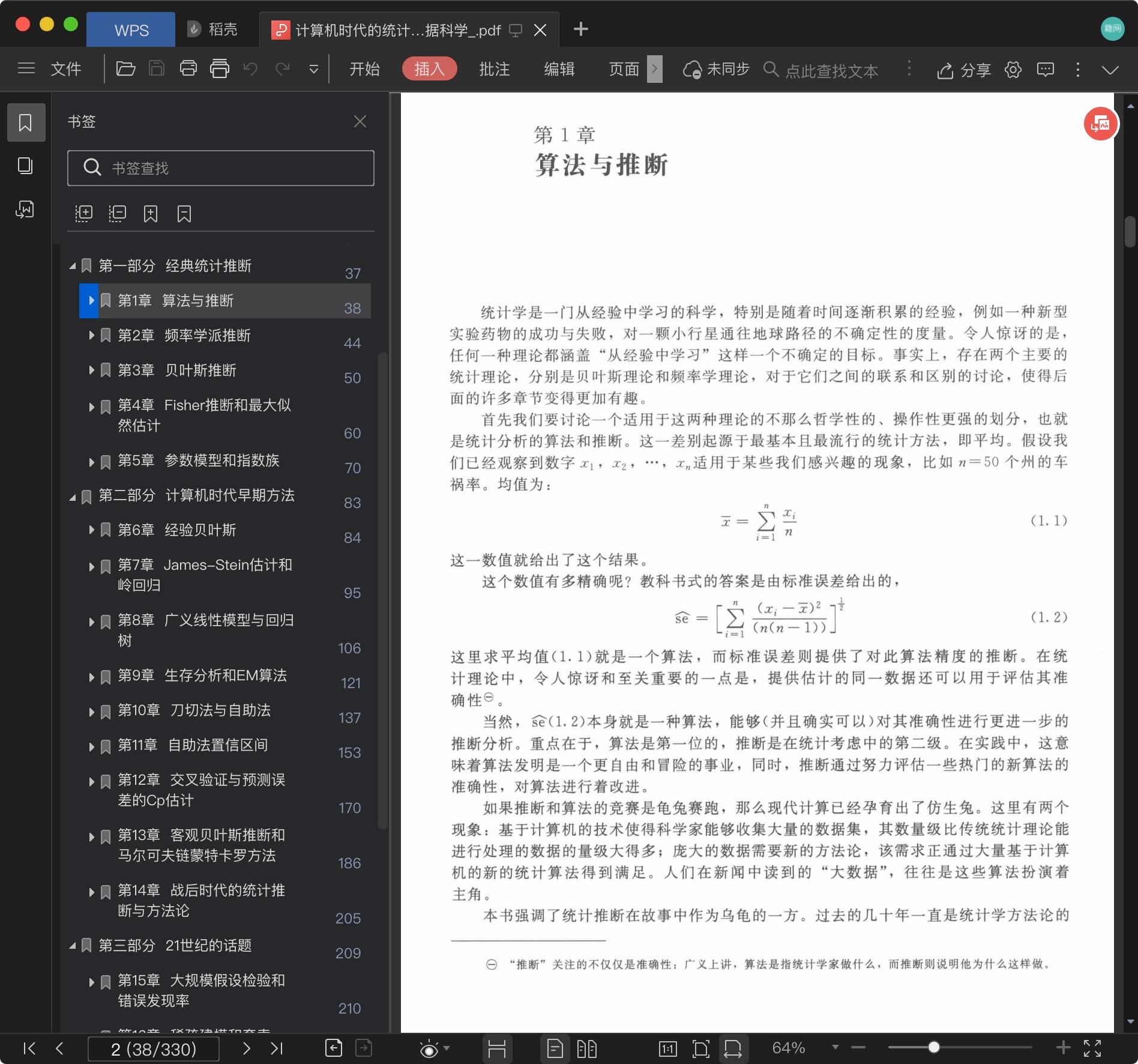
 
计算机时代的统计推断:算法、演化和数据科学pdf百度网盘下载地址?
本书以丰富的案例介绍了计算机时代下的统计推断的发展脉络,从理论的角度剖析统计推断的各类算法、证据等,揭示统计推断如何推动当今大数据、数据科学、机器学习等领域的快速发展并引领数据分析的变革,最后展望了统计学和数据科学的未来方向。
作者简介:
Bradley Efron is Max H. Stein Professor, Professor of Statistics, and Professor of Biomedical Data Science at Stanford University, California. He has held visiting faculty appointments at Harvard University, Massachusetts, the University of California, Berkeley, and Imperial College of Science, Technology and Medicine, London. Efron has worked extensively on theories of statist... Bradley Efron is Max H. Stein Professor, Professor of Statistics, and Professor of Biomedical Data Science at Stanford University, California. He has held visiting faculty appointments at Harvard University, Massachusetts, the University of California, Berkeley, and Imperial College of Science, Technology and Medicine, London. Efron has worked extensively on theories of statistical inference, and is the inventor of the bootstrap sampling technique. He received the National Medal of Science in 2005 and the Guy Medal in Gold of the Royal Statistical Society in 2014. Trevor Hastie is John A. Overdeck Professor, Professor of Statistics, and Professor of Biomedical Data Science at Stanford University, California. He is coauthor of Elements of Statistical Learning, a key text in the field of modern data analysis. He is also known for his work on generalized additive models and principal curves, and for his contributions to the R computing environment. Hastie was awarded the Emmanuel and Carol Parzen prize for Statistical Innovation in 2014.
目录:
第1章 算法与推断2
第2章 频率学派推断8
第3章 贝叶斯推断14
第4章 Fisher推断和最大似然估计24
第5章 参数模型和指数族34
第6章 经验贝叶斯48
第7章 James-Stein估计和岭回归59
第8章 广义线性模型与回归树70
第9章 生存分析和EM算法85
第10章 刀切法与自助法101
第11章 自助法置信区间117
第12章 交叉验证与预测误差的Cp估计134
第13章 客观贝叶斯推断和马尔可夫链蒙特卡罗方法150
第14章 战后时代的统计推断与方法论169
第15章 大规模假设检验和错误发现率174
第16章 稀疏建模和套索191
第17章 随机森林和提升207
第18章 神经网络与深度学习224
第19章 支持向量机和核方法238
第20章 模型选择后的推断250
第21章 经验贝叶斯估计策略266
点击下载